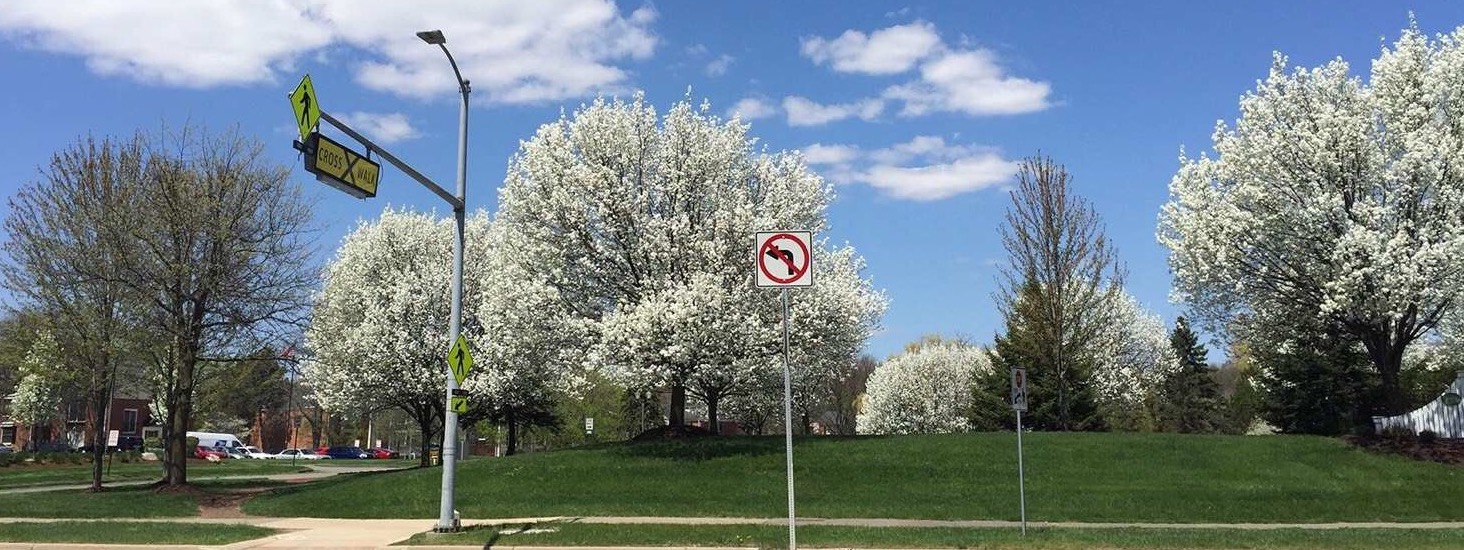
About
Bio
I am a Ph.D. candidate in Electrical and Computer Engineering department at Virgnia Tech. I'm honored to be advised by Prof. Lingjia Liu and have been fortunate to collaborated with Prof. Lizhong Zheng from MIT. My research interests include deep learning, machine learning, and their applications in wireless communication systems. .
Prior to join Virginia Tech, I received my M.S. degree in Electrical and Computer Engineering from University of Michigan, Ann Arbor and obtained my B.S. degree in Telecommunication Engineering from Beijing Institute of Technology.
News
[03/23/2024] Our paper "Learning at the Speed of Wireless: Online Real-Time Learning for AI-Enabled MIMO in NextG" has been accepted to IEEE Communications Magazine, 2024!
[11/20/2023] I gave a talk about our paper "2D-RC: Two-Dimensional Neural Network Approach for OTFS Symbol Detection" in Air Force Center of Excellence (CoE) meeting!
[10/24/2023] I passed my preliminary exam today!
[10/18/2023] I received IEEE MILCOM NSF Student Travel Grant!
[06/23/2023] Our paper "Detect to Learn: Structure Learning with Attention and Decision Feedback for MIMO-OFDM Receive Processing" has been accepted to IEEE Transcations on Communications, 2023!
[07/18/2022] I gave a talk about our paper "Learning to Equalize OTFS" in Air Force Center of Excellence (CoE) meeting!
[02/14/2022] Our paper "RC-Struct: A Structure-based Neural Network Approach for MIMO-OFDM Detection" has been accepted to IEEE Transcations on Wireless Communications, 2022!
[08/31/2021] I received the Prasad Scholarship Award from the Bradley Department of Electrical and Computer Engineering, Virginia Tech!
Selected Publications
More publications are in my Google Scholar page.
Jiarui Xu, Lianjun Li, Lizhong Zheng, Lingjia Liu. Detect to Learn: Structure Learning with Attention and Decision Feedback for MIMO-OFDM Receive Processing. Accepted to IEEE Transactions on Communications (TCOM), 2023. (Journal)
Jiarui Xu, Zhou Zhou, Lianjun Li, Lizhong Zheng, Lingjia Liu. RC-Struct: A Structure-based Neural Network Approach for MIMO-OFDM Detection. Accepted to IEEE Transactions on Wireless Communications (TWC), 2022. (Journal)
Lianjun Li, Jiarui Xu, Lizhong Zheng, Lingjia Liu. Real-Time Machine Learning for Multi-User Massive MIMO: Symbol Detection Using Multi-Mode StructNet. Accepted to IEEE Transactions on Wireless Communications (TWC), 2023. (Journal)
Zhou Zhou, Lingjia Liu, Jiarui Xu, Robert Calderbank. Learning to equalize OTFS. Accepted to IEEE Transactions on Wireless Communications (TWC), 2022. (Journal)
Zhou Zhou, Lingjia Liu, Jiarui Xu. Harnessing Tensor Structures —- Multi-mode Reservoir Computing and Its Application in Massive MIMO. Accepted to IEEE Transactions on Wireless Communications (TWC), 2022. (Journal)
Chen Gao, Jiarui Xu, Yuliang Zou, Jia-Bin Huang. DRG: Dual Relation Graph for Human-Object Interaction Detection. Accepted to European Conference on Computer Vision (ECCV), 2020.
Academic Services
Journal Reviewer
IEEE Transactions on Wireless Communications (TWC), 2024.
IEEE Journal of Selected Areas in Communications (JSAC), 2023.
IEEE Transactions on Neural Networks and Learning Systems (TNNLS), 2021, 2022.
IEEE Transactions on Vehicular Technology (TVT), 2023.
Journal of Visual Communication and Image Representation (JVCI), 2022.
Conference Reviewer
IEEE Global Communications Conference (GLOBECOM), 2023
Letter Reviewer
IEEE Communications Letters, 2024.
Contact Me
Feel free to contact me at jiaruixu [at] vt [dot] edu